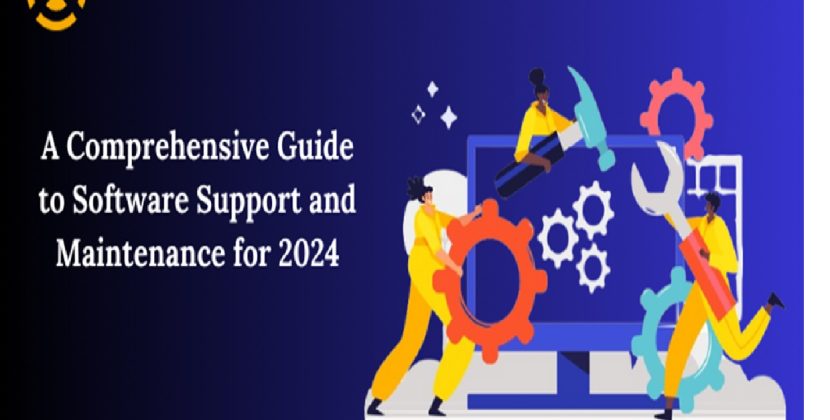
AI Brings The Best In CyberSecurity
Security is a continual worry for businesses, and most have begun to use threat detection software rather than depending solely on the manual effort of software developers and cybersecurity specialists. TDS (thread detection software) and SIEM (security information and event management) solutions are used by high-performance enterprises to discover issues before they spread and cause damage.
When a network or security problem occurs in an organization, every second matters, since even a minute of outage can cost thousands of dollars.
Artificial intelligence (AI) is seen as a possible approach for improving threat detection and reducing alert fatigue, which can paralyze NOC and SOC staff. Early adopters of machine-learning algorithms have seen a 90 percent drop in the number of alarms and a significant gain in efficiency. EPP, SOAR, and NTA are all possible applications for this new technology.
In the field of cybersecurity, data analysis is not a new concept. It has already been utilized as a SIEM tool, but classifying alerts, creating tickets, and managing reports manually requires a complete crew to labor around the clock. Unfortunately, most businesses cannot afford a unit capable of analyzing all of the signals created by the automated systems they employ.
Cybersecurity alerts and IT automation
Automated systems can be used to analyze most organizational processes. However, when compared to real threats, these have a relatively high rate of false positives.
The main reason for this is that most automated network monitoring solutions focus on single event matching rather than contextual analysis. Simply said, if the computer finds a single abnormality, it will issue an alert without questioning why it occurred or whether it is a genuine threat. It’s preferable to be overwhelmed than to be sorry. ZIP archiving, which is regarded as ransomware because of its identical read/write behavior, is an outstanding example.
Filtering out unimportant signals by giving priorities is how cybersecurity AI decreases alert noise. Auto-closure of false positives or low-value warnings, auto-association of alerts triggered by the same event, and auto-escalation of situations needing human help are all capabilities of a resilient system. In the latter circumstance, some explanation, such as an error code, is connected to the situation.
How can AI sort false positives out of tons of alerts?
Alert triage based on contextual information and event connection is the key to combating alert fatigue. Artificial intelligence must replace the hours or even days of detective work undertaken by security specialists with automated replies delivered in seconds or minutes.
The best approach to do this is to do a root cause analysis and trace the chain of events that led to the alert, looking for repercussions (backward and forward tracking). When you consider the context, it’s easier to tell the difference between suspicious procedures and serious threats.
This study would require a cybersecurity expert to examine a graph with hundreds of consequences for each alarm. This task would just add to the problem’s complexity and length of time to solve. Thousands of alternatives are assessed continuously and instantly using big data when manual work is AI-based. Each occurrence is compared to a database of comparable occurrences that are categorized by rarity. The distinction between single event solutions and multi-event solutions is that when analyzing the impact and possibility for that alert to become a real-life detrimental event, the whole chain of causality is considered.
When a company is attacked, every minute counts, and the hours spent cleaning the system and restoring it to its original state can cost millions of dollars. In this instance, one of the most important KPIs is the time it takes to figure out what caused the problem.
Enabling performant alert triage with AI
Using AI to enable efficient warning triage at the enterprise level entails screening out threats while keeping a proper level of alertness. To avoid system paralysis, top firms are dealing with an alert overload and need to limit noise notifications.
They require precise alerts that are only escalated after being vetted by numerous automated systems and determined to be dangerous, and there is no standard protocol in place for that situation.
Big data is used by AI technologies like Arcanna.ai alert triage to imitate the difficult triage process used by security specialists. The machine learning system examines millions of data points to determine the context of each warning, which is then categorised using NLP (Natural Language Processing) on the alert text as well as learnings based on analyst comments. Because their pattern does not match the pre-existing database, such a system should produce less than 1% of occurrences that are not automatically categorized.
So, is there a future for AI in CyberSecurity?
Certainly. False positives consume a significant amount of time for businesses. The approach is to use a program that distinguishes between malware and real-world threats. The objectives include dramatically lowering false positives, providing context for each event, and aggregating several notifications relating to the same occurrence.
With the advent of IoT, choosing a machine-learning-based solution will become the only realistic option, as alerts will increasingly be defined by the three Vs. of big data: volume, velocity, and variety.